OpenGroove
Summary
For my thesis, I taught a machine to dance. I used LSTM RNNs (Recurrent Neural Networks) to “learn” the relationship between human joint movements and music. With the help of Google‘s open-source pose estimation API, I was able to extract “dance data” from solely videos of my colleagues having a boogie. Previous state-of-the-art AI dance has required very expensive motion capture studio set ups to collect this dance data - my method negates this requirement and hence makes this early-stage art form more accessible to researchers, artist and engineers.
Objectives
1. Create an entirely open-source method of AI dance synthesis, without the need of expensive motion capture data.
2. Explore and test engagement factors associated with AI dance in VR concert environments.
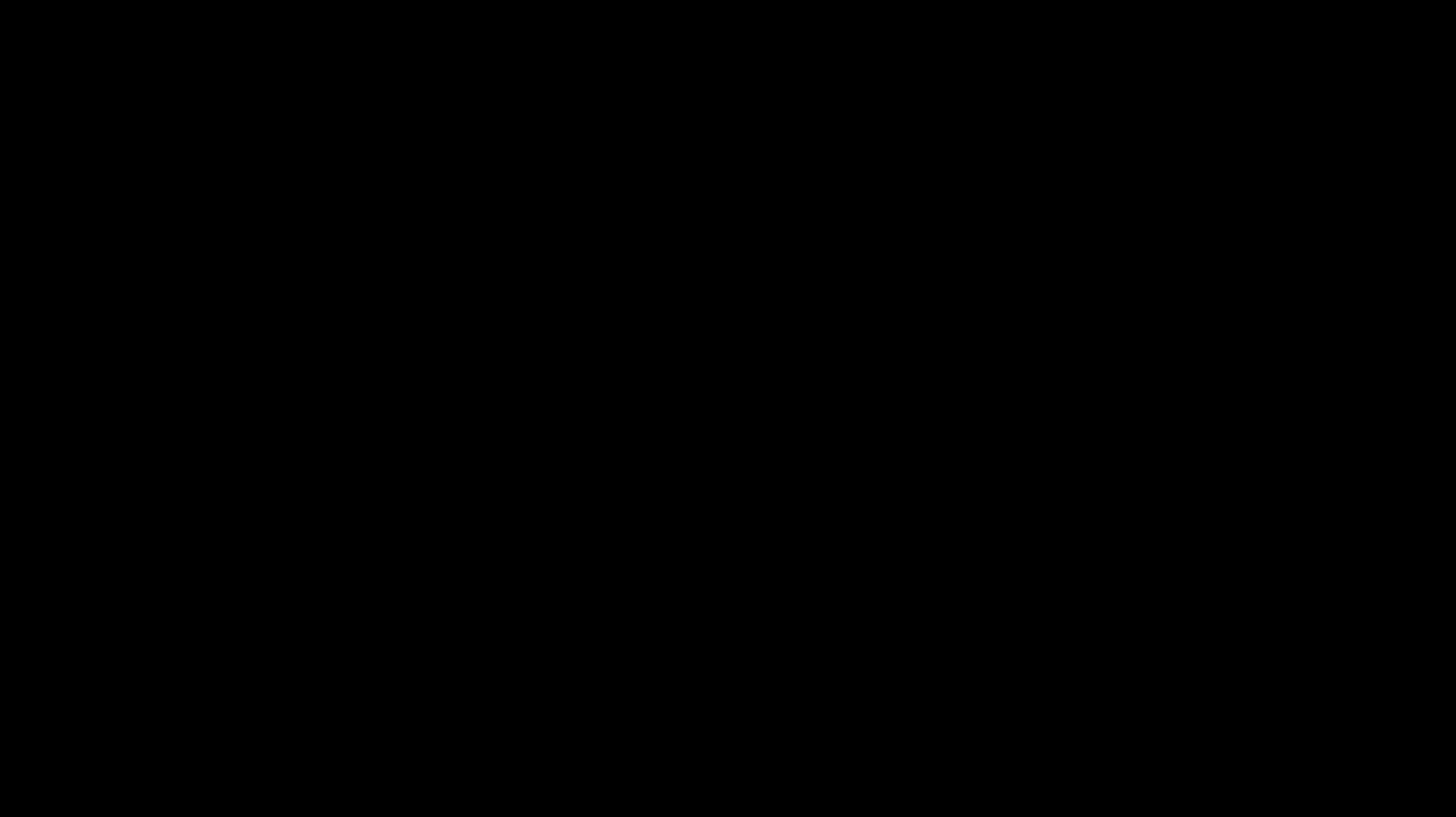
Collecting joint movement data
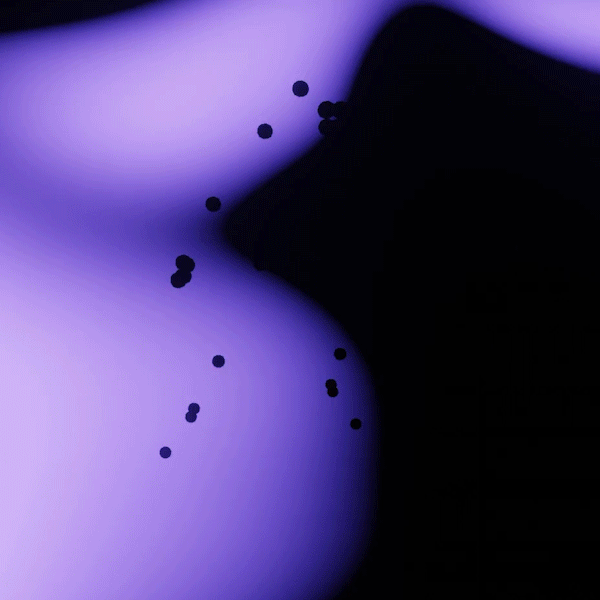
AI Dance Output (Blender)
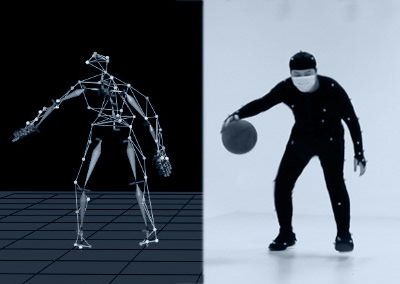
Motion Capture: An expensive method of dance data collection.
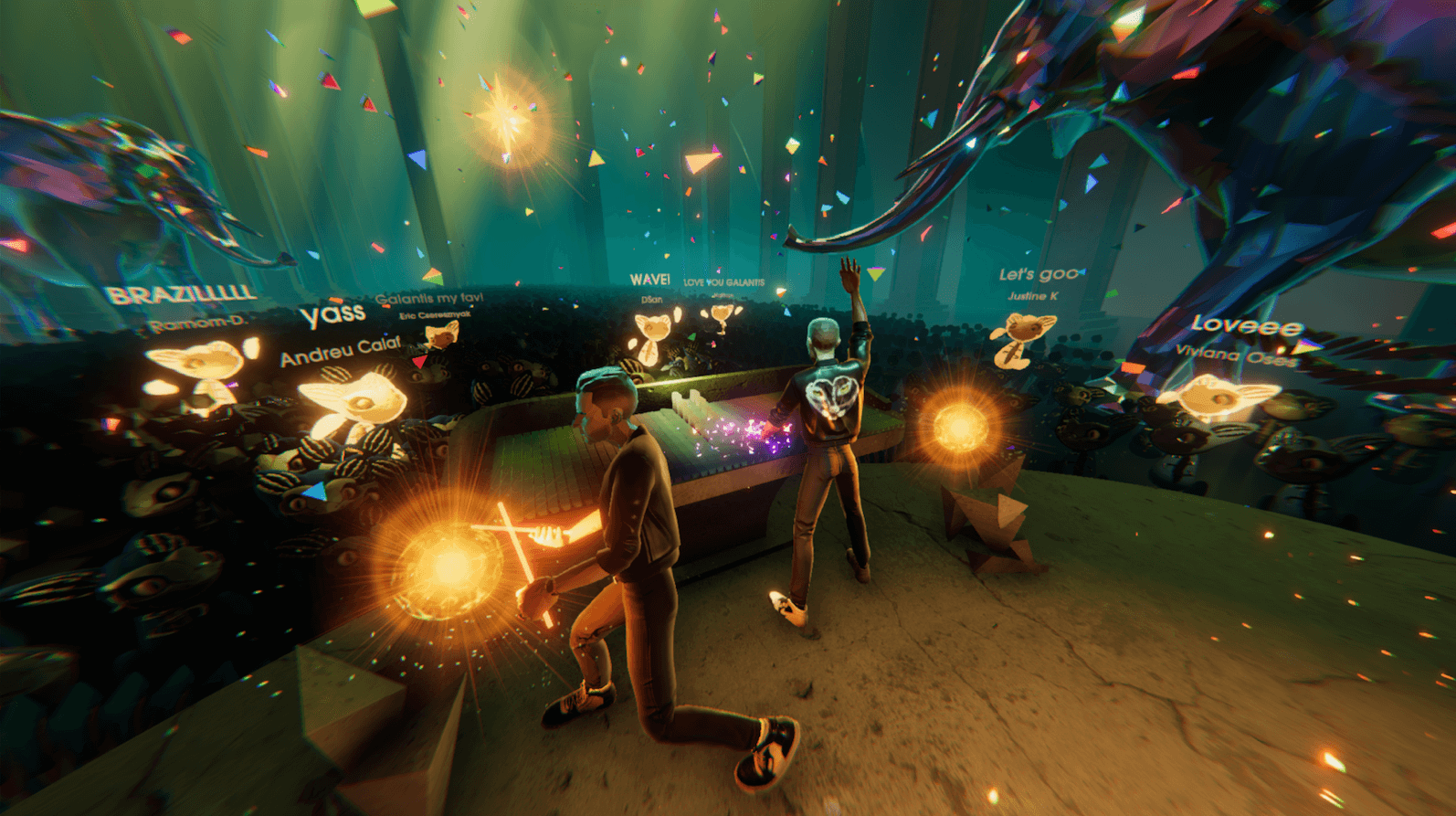
Contemporary VR concert environment
Dance Data Collection
Mediapipe, Google's open source human pose estimation library, was used an alternative method of dance data collection to Motion Capture. This powerful library allowed the 3D coordinate tracking of all human joints. This drastically reduced the cost of cost of data collection - as expensive motion capture studios were no longer required.
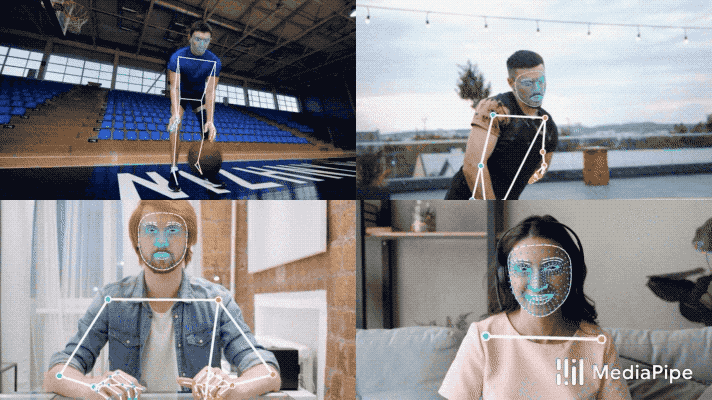
MediaPipe - Google's Human Pose Estimation API
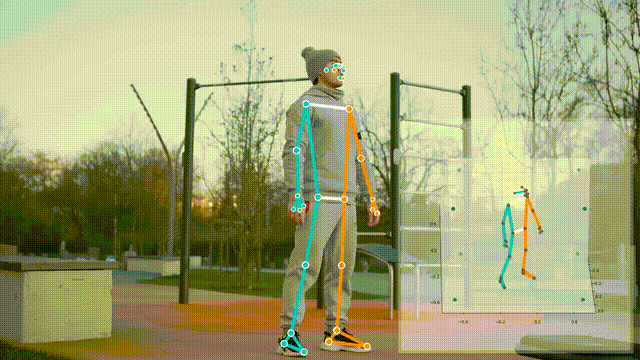
Music Data Collection
During dance data collection, audio features were extracted using Essentia, an open-source C++ library. A total of 69 audio features were extracted for each frame of dance and paired with joint movement data.
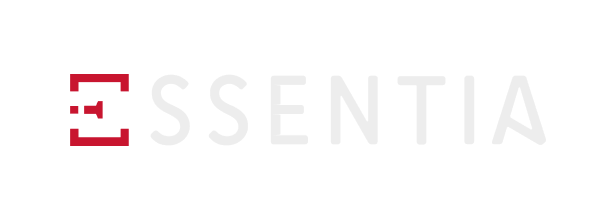
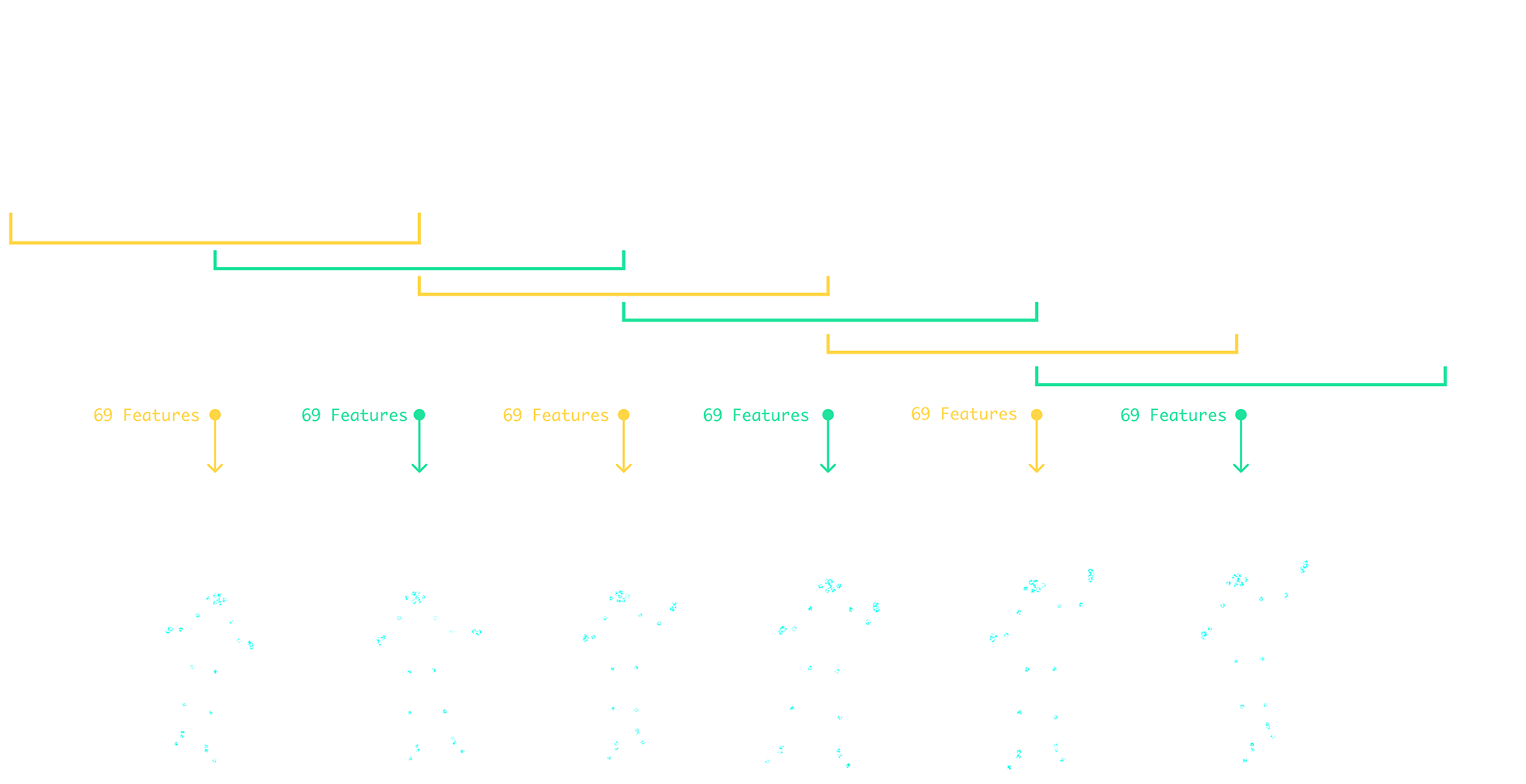
The Machine Learning Process
Machine learning models were developed to learn the relationship between music and dance patterns. Three variations of LSTM-RNN (Recurrent Neural Networks) were used, exploring different dimension reduction techniques to improve dance output. The models aimed to generate dance when given unfamiliar music.
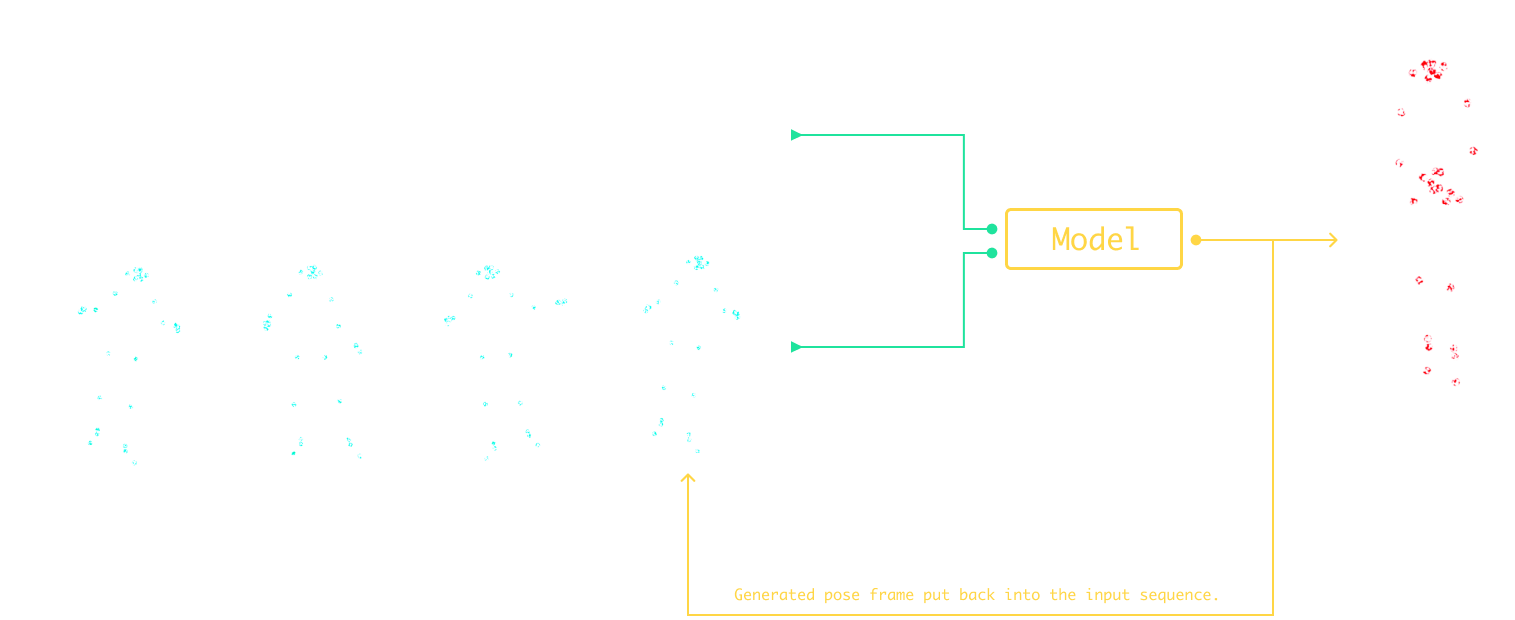
The models learns the relationship between music and dance.
Model Comparison
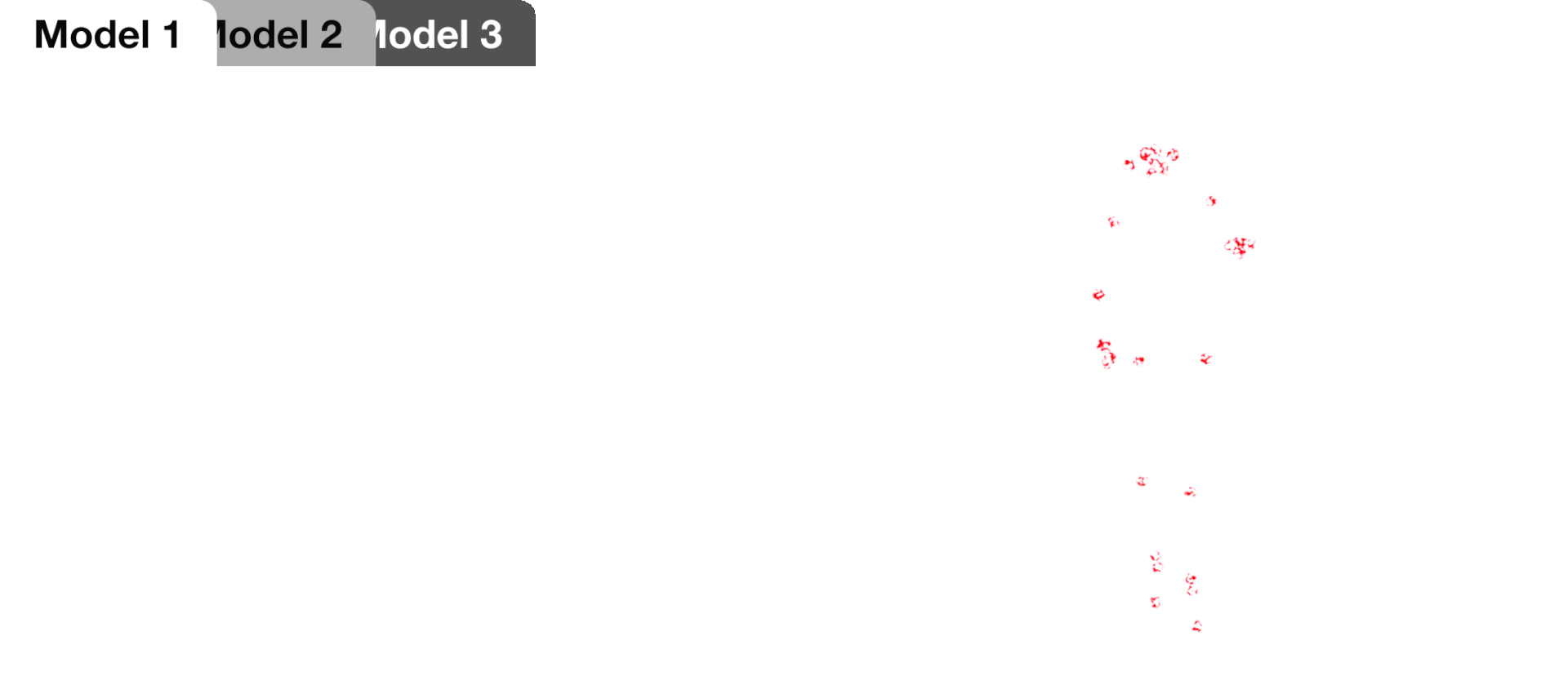
Model 1 was a 3-layer encoder-decoder LSTM-RNN used as a baseline. It ensured correct transformation of features and labels and served as a starting point for further development. The encoder-decoder architecture involved the first LSTM layer converting the input sequence to a fixed-length context vector, enhancing subsequent LSTM layers' forecasting ability.
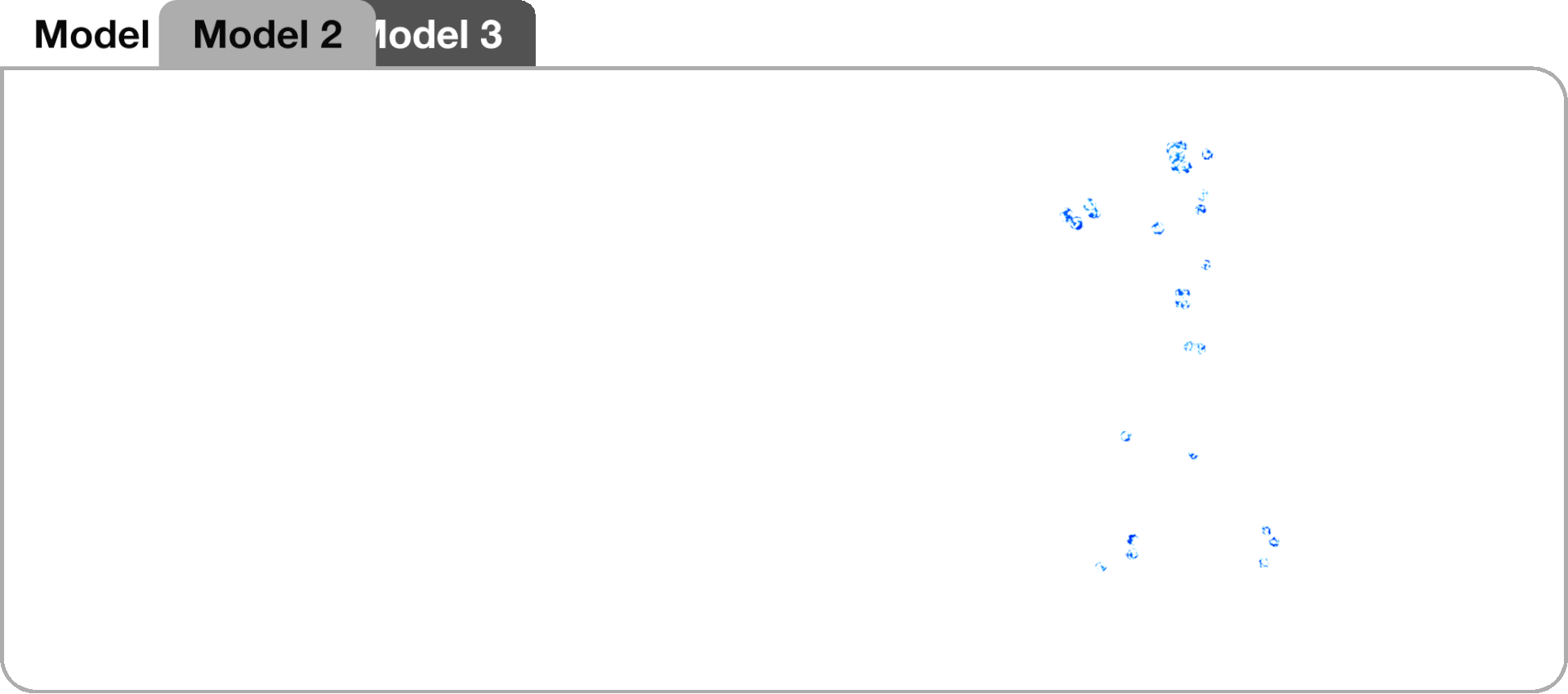
Model 2, an extension of the baseline, utilized a CNN-LSTM encoder-decoder architecture inspired by successful audio-driven motion synthesis applications. Instead of an LSTM layer, two 1-dimensional convolutional layers (Conv1D) were used as the encoder. The Conv1D layers extracted features, which were then pooled and flattened before being passed to the LSTM layers
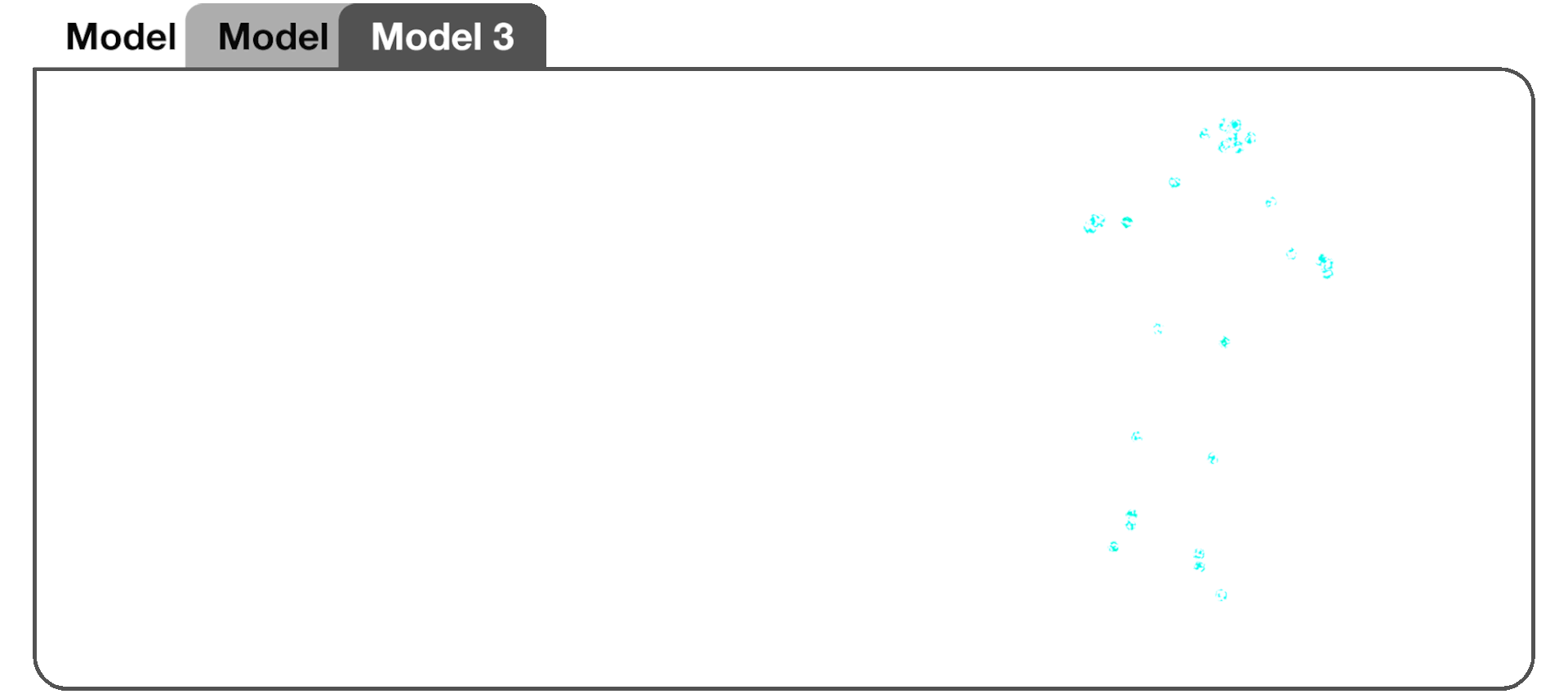
Model 3, an extension of the CNN-LSTM encoder-decoder, introduced a multi-head architecture. Multiple sets of Conv1D layers (heads) were used to independently extract convolved features for each time series. This allowed each of the 168 features to have its own dedicated set of Conv1D layers or "heads".
Dance Visualisation in Blender
Animation from model output:
Blender, a free open-source 3D graphics software, was used to visualize the generated pose sequences from the CSV files. Using the embedded Python interpreter within Blender, mesh objects representing the 33 human joint positions were created, and animation keyframes were generated for each pose sequence. A speaker object was added to play the corresponding audio file. Different camera angles and defamiliarization techniques, such as tracking shots, panning shots, static shots, tilt shots, and close zooms, were applied to create a dynamic dancing sequence. The Blender animation framerate was set to 30 FPS to ensure synchronization with the audio
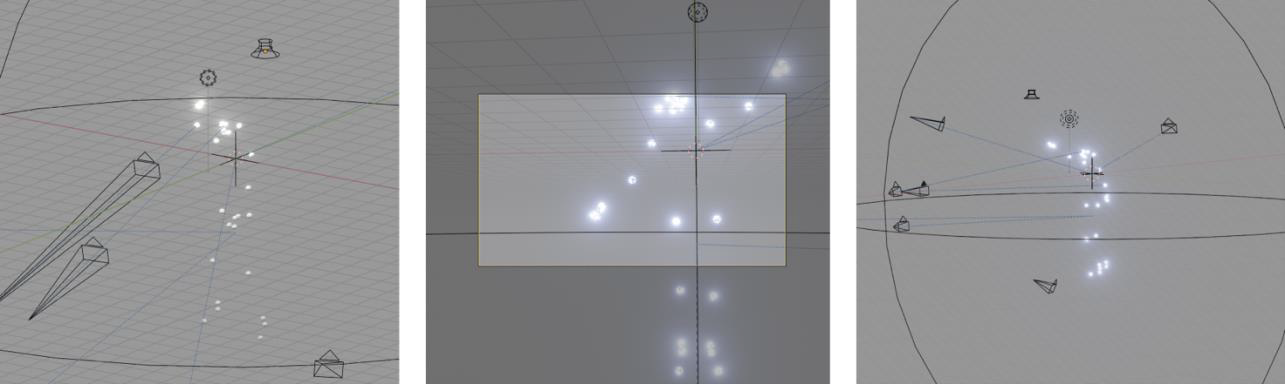
Blender scene configured with different camera angles / filmic techniques reviewed in literature
Model Evaluation
Quantitative:
After training, the mean squared error (MSE) losses of each model were recorded, and their regression fit was analyzed. Model 2 showed significantly lower losses compared to models 1 and 3, with a reduction of approximately three times. None of the models were able to generalise to test data of – however, further exploration is required test song choices.

Model Performance Metrics (Quantitative)
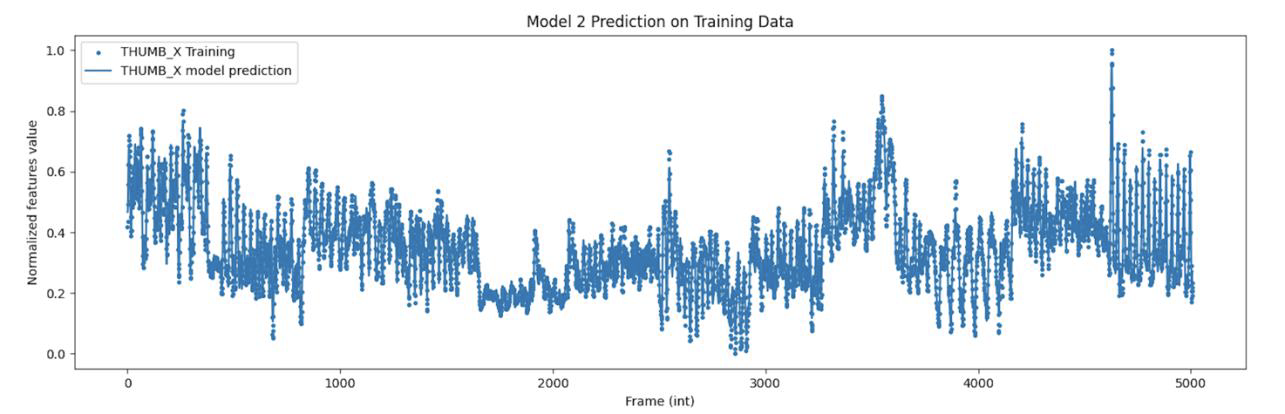
Model 2 prediction with respect to training data.
The generation process in dance synthesis involves forecasting each pose frame independently and feeding it back into the input sequence iteratively. This iterative process can compound errors and lead to differences between the generated pose sequences and the training data. These differences result in the generation of novel dance sequences, as shown below. It is important to note that while mean squared error (MSE) loss or other performance metrics are useful, they may not capture the full essence of dance synthesis and should be considered alongside other factors.
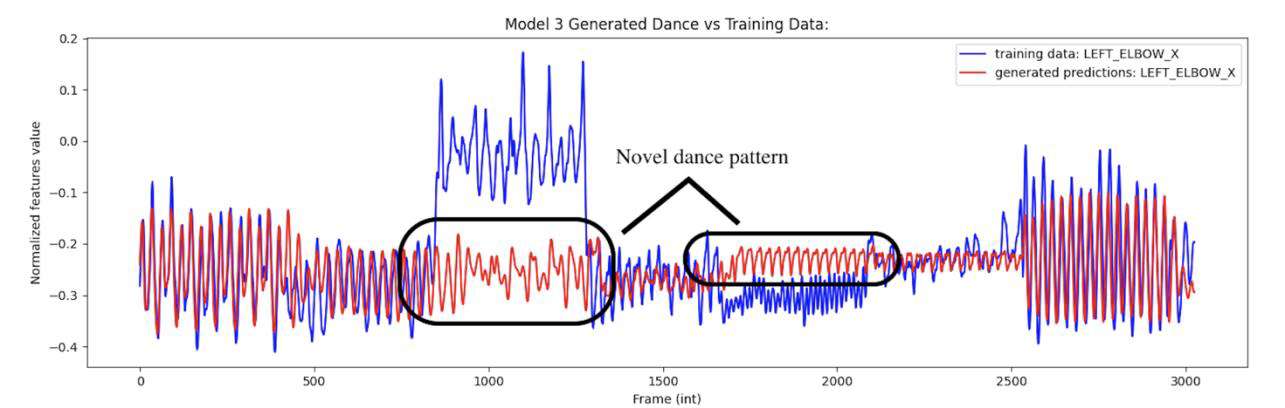
Generated dance sequences with respect to original training data
Qualitative:
Participants rated Model 2, the CNN-LSTM, as the best performer. It successfully learned almost all the dancing patterns from the training data and was perceived as more expressive and energetic in its dance choreography compared to the other models.

Qualitative user scores for model evaluation on dance fidelity and synchronicity.
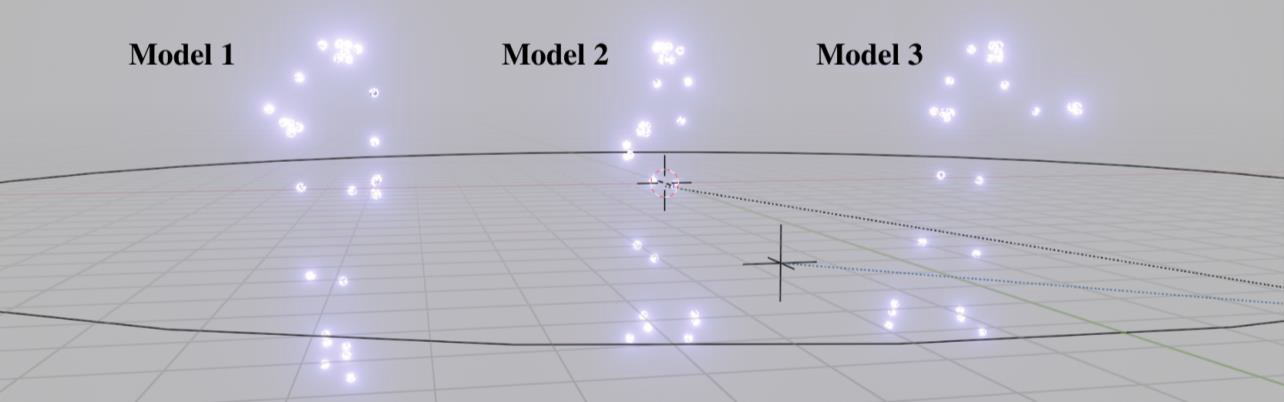
3 models visualized, each producing their own unique dance pattern at a given frame.